As we step into the intricate landscape of advertising technology, the role of Natural Language Processing (NLP) emerges as a cornerstone in understanding and leveraging the emotional and intellectual engagement of audiences. This approach, focusing on sentiment analysis, topic modeling, and the precision tools of keyword extraction and named entity recognition, offers a comprehensive view into the mechanics of ad content strategy. It's about capturing the pulse of consumer sentiment, refining marketing messages to resonate more deeply with target audiences.
Understanding Sentiment Analysis in Ad Content
Sentiment analysis through Natural Language Processing (NLP) fundamentally revolutionizes ad content strategy by tapping into the emotional feedback of customers. This tool scrutinizes text from reviews, social media, and other sources, identifying whether the tone is positive, negative, or neutral. By deciphering the emotional undertones in customer opinions, businesses harness the power to tailor their marketing strategies in real time, enhancing relevance and engagement.
At its core, sentiment analysis reveals what customers feel about products or brands, beyond mere satisfaction levels. Positive feedback uncovered by NLP might highlight the strengths of a product, encouraging companies to emphasize these aspects in future ads. Conversely, detecting widespread negative sentiments could alert businesses to potential issues or unfavorable perceptions that need addressing, either in the product itself or in how it's presented.
NLP processes information at an impressive scale and speed unmatched by manual methods. This rapid processing capability allows companies to remain agile, updating their ad content strategies in alignment with current consumer sentiments. It's like having your finger on the public's pulse, ready to adapt messaging at a moment's notice to maintain a strong connection with your audience.
Sentiment analysis aids in crafting personalized ad experiences. Understanding the emotional nuances of different demographic segments allows marketers to modify their strategies to speak directly to varied audience feelings and preferences. It's akin to choosing a gift that resonates precisely with someone's likes.
The technology excels in pinpointing emerging trends and shifting sentiments about a brand or product. Marketers can capture subtle shifts in public opinion, enabling them to either ride the wave of growing positive sentiment or counteract negative trends before they damage brand reputation.
Another intriguing application is competitor analysis. By applying sentiment analysis to what people are saying about competitors' products and ads, companies gain insights into rivals' strengths and weaknesses. This intelligence informs strategic decisions, providing an edge by learning from others' successes and missteps.
Finally, by analyzing sentiment over time, businesses track the long-term impact of their ad content strategies. They observe how changes in messaging influence public perception, refining their approach based on clear patterns emerging from the analysis.
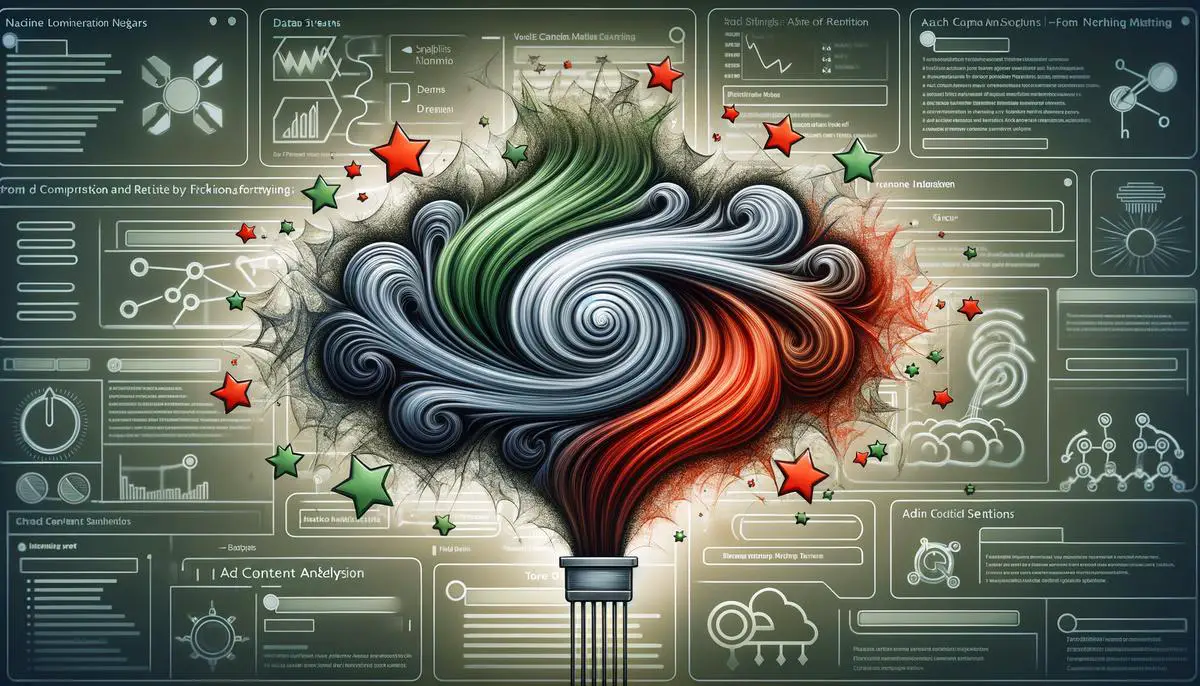
The Role of Topic Modeling in Content Strategy
Topic modeling acts as a powerful tool in the toolbox of data scientists and marketers, sifting through heaps of text to uncover underlying themes coursing through ad materials. It's akin to having a high-powered sieve that separates the wheat from the chaff. Here's a breakdown of how it breathes life into ad content analysis:
- Topic modeling scans through ad content, not just for what's explicitly said but for the latent topics simmering beneath. These might be trends stirring interest or niches just coming into play.
- The process introduces marketers to clusters of related words or phrases signaling certain topics. These clusters serve as gold mines for understanding what resonates with the audience.
- With topic modeling lighting the way, advertisers can spot patterns early and channel their efforts into creating content that hits these interests head-on.
- The advantage of using topic modeling spans beyond just surfacing these gems. It informs a could-be blurry picture of customer behavior and shifts the needle from wide-net strategies to sharply targeted campaigns.
- By tailoring ad campaigns to reflect prominent and emergent topics unearthed by topic modeling, companies not only stand out in a sea of ads but resonate with their audience on a deeper level.
- Topic modeling's bounty of insights enables marketers to pivot quickly. This agility is critical in the ever-shifting quicksand of consumer interests and market trends.
- In fine-tuning ad content with insights derived from topic modeling, there's also a delightful side effect: audience learning. Through focused content, brands educate their audience about hitherto unexplored areas aligned with emerging interests, helping forge a stronger, more informed connection.
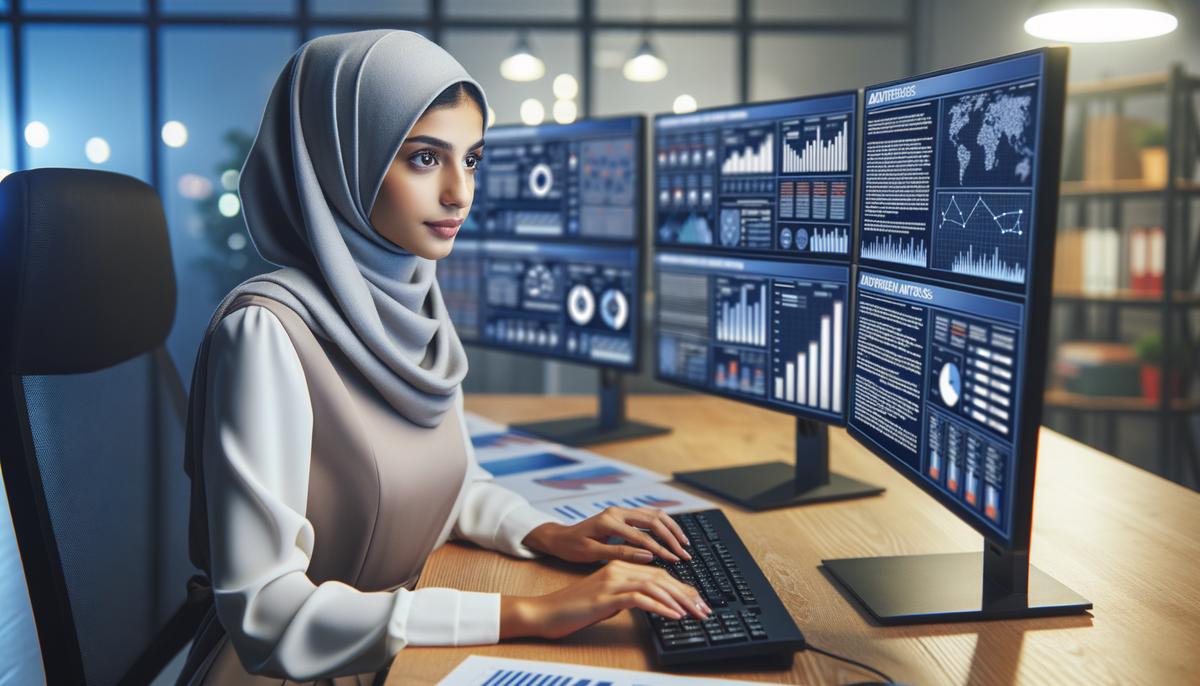
Keyword Extraction and Named Entity Recognition
Keyword extraction discerns the primary subjects or themes within your ads. This technique pulls out words or phrases deemed crucial, offering direct insight into the ad's focus, like lifting out gems from a bed of gravel. It's pivotal for advertisers aiming to ensure their content is honed to perfection, targeting the right audiences with the right words at the right time.
Named entity recognition (NER) carves out the 'who' and the 'where' – pinpointing people, companies, geographical locations, and other specified categories nestled within the ad content. NER supplies you with hi-tech binoculars, allowing you to spot and catalog the critical entities dancing through your text. This precision tool doesn't just highlight but categorizes entities, crucial for ads aiming to relate personally to its viewers or leverage topical, location-based strategies.
Combining these twin titans of NLP in ad content analysis redefines accuracy. By identifying both pivotal keywords and entities, ads can be crafted to cut sharper, deliver messages that resonate more deeply, and ultimately thread a tighter connection with potential consumers. Whether zeroing in on trend-driven keywords or spotlighting entities that anchor your brand's message, these techniques empower ad strategies to not merely reach but truly engage their intended audiences, turning bystanders into buyers.
Employing keyword extraction and NER reimagines ad content design. It propels inventory checks on which terms and entities you're hedging your bets on and recalibrates your approach based on solid data-driven insights. Imagine releasing ads into the wild and having them magnetize the right eyeballs because every word aligns perfectly with what your audience seeks or values. The outcome? A study by Wordstream found that using relevant keywords in ad copy can increase click-through rates by up to 15%.1 Enhanced click rates and a richer, more purpose-driven audience interaction.
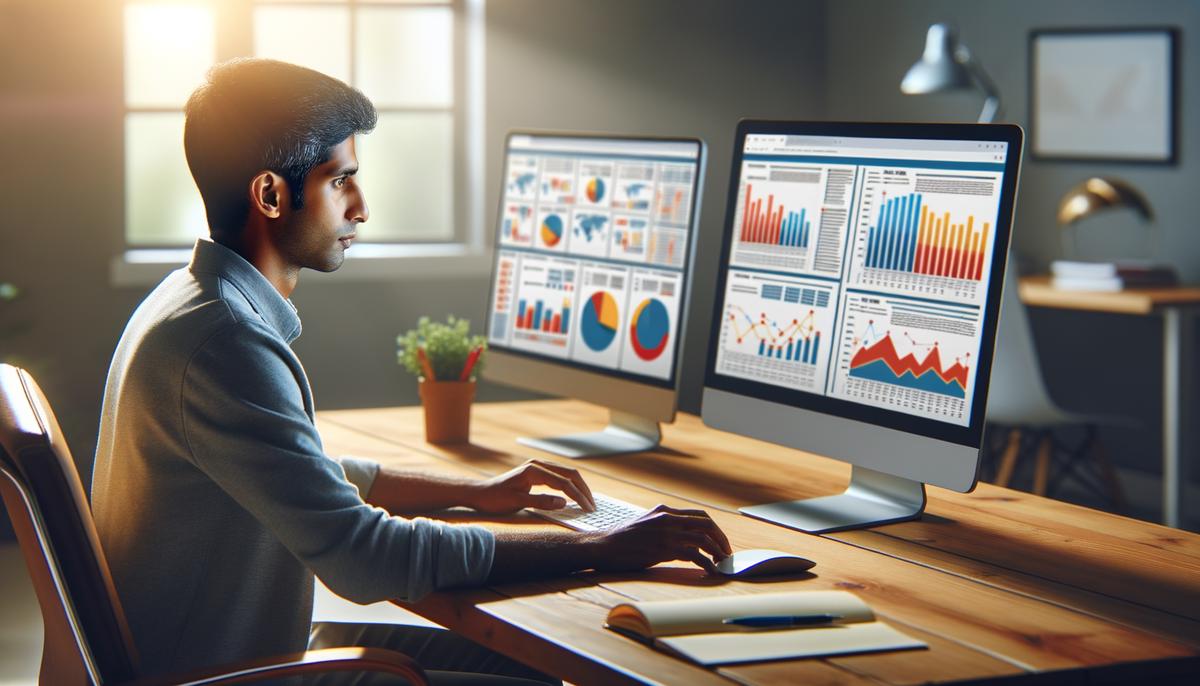
Challenges in NLP for Ad Content Analysis
Navigating the humongous volume of data that digital advertising campaigns generate presents a significant challenge in applying NLP. Every click, view, share, or comment becomes a data point, culminating in a dataset as vast as the digital universe itself. Sifting through this digital cosmos to extract meaningful patterns requires state-of-the-art computing power and advanced NLP algorithms. Picture trying to count every star in the sky—now imagine trying to understand each star's story.
The complexity of the data itself further complicates NLP application in ad content analysis. Just as human language is layered with nuance, humor, and irony, so too is the language of ads multifaceted. Interpreting sarcasm, cultural references, or subtleties within ad content goes beyond mere keyword analysis, demanding a keen AI trained on diverse linguistic datasets. It's like trying to understand an inside joke at a party—all the context in the world won't matter if you don't know the backstory.
Advanced algorithms are crucial for parsing through this complex, voluminous data effectively. These algorithms need to be both powerful and nuanced, capable of distinguishing between context-driven sentiment and literal word meaning—a process complicated enough that it's akin to translating foreign poetry where every word has multiple layers of meaning.
Striking a balance between leveraging NLP for actionable insights and maintaining user privacy and data security adds another layer of difficulty. As digital footprints expand, so does concern over confidentiality and misuse of personal information. In a survey by Pew Research Center, 79% of Americans reported being concerned about how companies use their data.2 Ensuring that AI's analytical prowess doesn't infringe on individual rights is like walking a tightrope over the Grand Canyon—awestruck by the potential view but acutely aware of the need for balance.
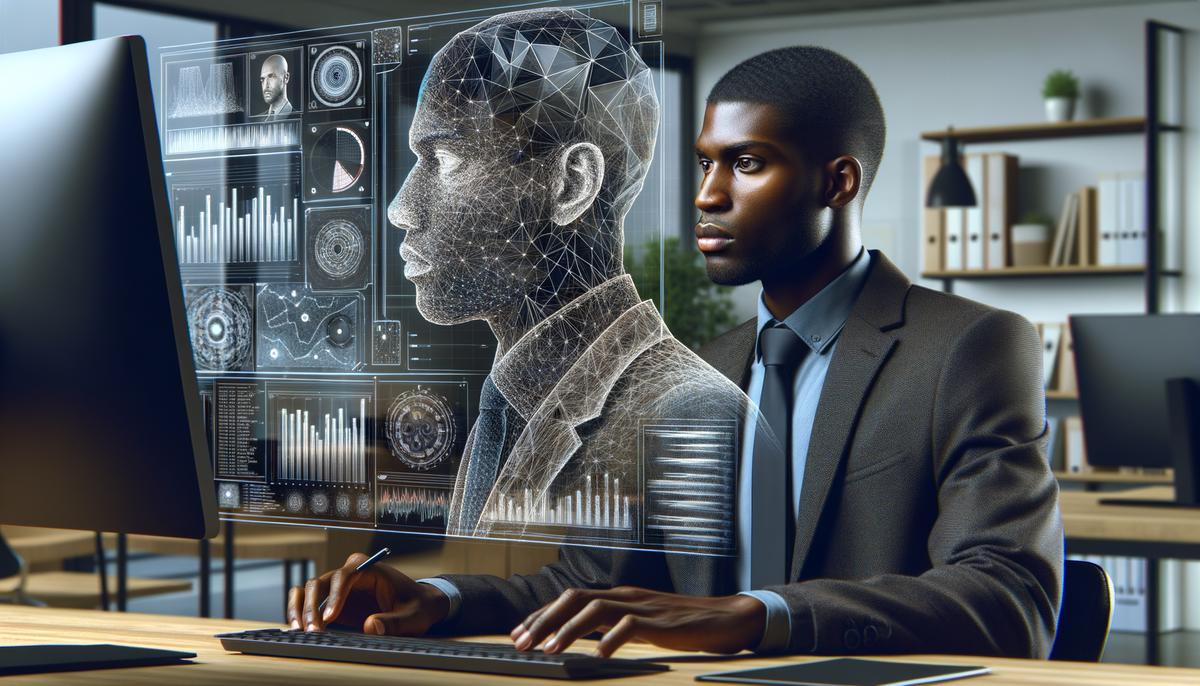
The Future of NLP in Advertising Technology
As the digital horizon expands, the integration of voice and visual search into advertising sets the stage for a transformative shift. Picture an advertising platform that seamlessly responds to spoken queries or visual cues, making product discovery as natural as talking to a friend. This evolution requires NLP systems to accurately interpret the nuance of human language and the context of images, further blurring the lines between digital interaction and human conversation.
The synthesis of NLP with augmented reality (AR) in advertisements could allow customers walking past a store to receive real-time, AR-enhanced ads on their mobile devices, personalized through voice commands or visual prompts. This leap would not only personalize shopping experiences but also anchor brand messages in memorable, interactive moments.
Generative AI models, an offshoot of sophisticated NLP applications, promise to automate and optimize ad copy generation. By analyzing successful ad campaigns and ongoing consumer conversations, these models could generate creative, targeted ad content, reducing the creative burden on human marketers and ensuring a higher resonance with targeted audiences.
Progress in machine learning algorithms will revolutionize predictive analytics in advertising. These algorithms, fed with vast datasets analyzed through NLP, could predict consumer trends and responses to particular ad content with unprecedented accuracy. Marketers could then anticipate shifts in consumer sentiment and adjust campaigns proactively, staying always a step ahead.
Ethical AI use will become a cornerstone of future advertising strategies, reflecting growing consumer awareness and regulatory pressures about data privacy and security. Advertisers employing NLP must navigate these waters carefully, ensuring transparency and the responsible use of consumer data to build trust and loyalty, rather than suspicion.
Cross-lingual NLP capabilities will break down language barriers, opening new markets for brands. By effortlessly analyzing and generating ad content in multiple languages, businesses can connect with diverse audiences worldwide, tailoring messages to cultural nuances without losing brand consistency.
Collaborative filtering, underpinned by NLP, will refine recommendation engines, ensuring that advertised products match the viewer's preferences and prior behavior with uncanny precision. Amazon's recommendation engine, powered by collaborative filtering, is responsible for 35% of the company's revenue.3 This not only enhances user satisfaction but also increases the likelihood of conversion, directly benefitting the bottom line.
The integration of NLP in customer service bots will ensure that post-ad interaction remains engaging and helpful, turning customer service from a cost center into a powerful extension of the advertising strategy. As these bots become more adept at understanding and responding to complex queries, the customer journey from ad impression to purchase becomes seamless and frictionless.
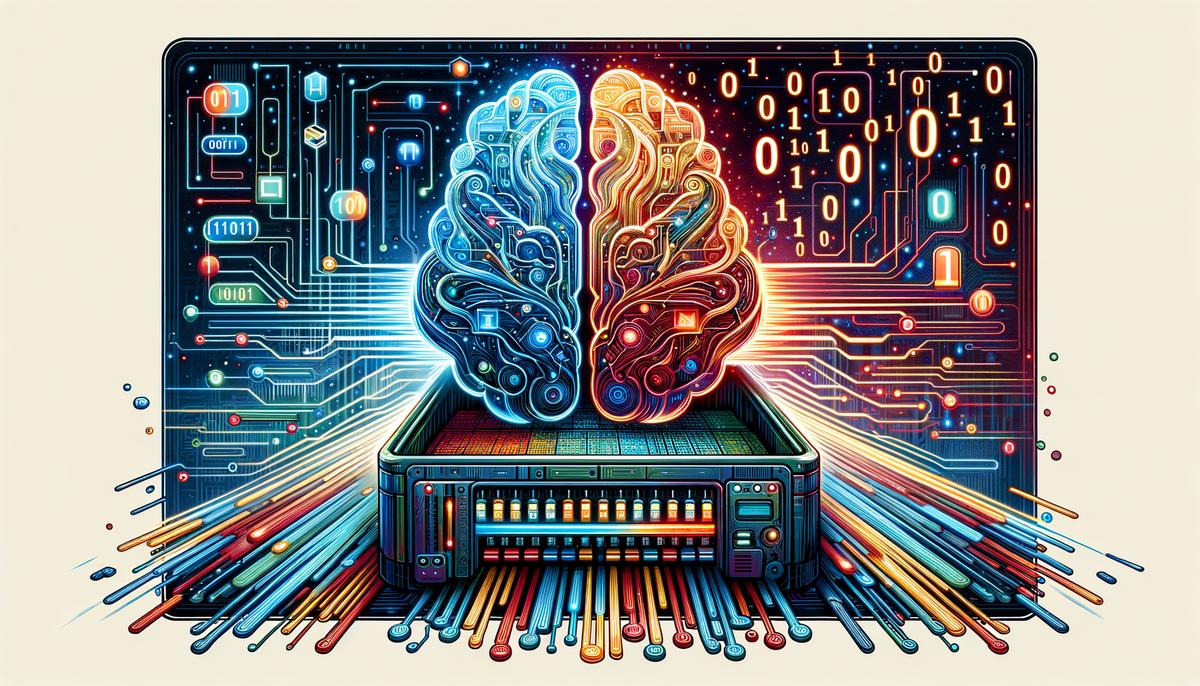
The essence of integrating NLP into advertising strategies lies in its ability to transform vast datasets into actionable insights, fostering a deeper connection between brands and their audiences. By continuously refining and personalizing ad content based on real-time analysis, businesses can maintain a dynamic and engaging dialogue with consumers, ensuring that every campaign not only reaches but truly speaks to its intended audience.
- Laubheimer P. The Role of Keyword Choices in the User Experience. Nielsen Norman Group. Published March 25, 2018.
- Auxier B, Rainie L, Anderson M, Perrin A, Kumar M, Turner E. Americans and Privacy: Concerned, Confused and Feeling Lack of Control Over Their Personal Information. Pew Research Center. Published November 15, 2019.
- MacKenzie I, Meyer C, Noble S. How retailers can keep up with consumers. McKinsey & Company. Published October 1, 2013.